How a Top 3 Pharmaceutical Company Stopped Spending Time Manually Ingesting and Analyzing R&D Data
Written By:
Brendan Guerin

Imagine you are a data science lead at a pharmaceutical company. Your organization has invested millions in data assets (in terms of time, money, expert labor). You’ve spent years carefully curating, harmonizing, and enriching the data. You’ve stood up a powerful internal knowledge graph that, in principle, should empower your organization to make better, data-driven decisions when it comes to critical tasks like target identification and prioritization. Yet only a tiny fraction of the information is actually being used by anyone.
Can you feel the frustration? Can you imagine the cost of all that wasted effort? The cost of all those wasted hours and resources?
This was the exact situation of our client. They were sitting on an immensely valuable knowledge graph with a team of smart data scientists ready to analyze it–yet they found that it was still too time consuming to extract insights. The problem wasn’t the data, it was the tools they had to leverage it. They simply weren’t fast enough.
When internal stakeholders asked the data science team for a specific analysis, they had to manually pull the data, code up Jupyter notebooks and data visualizations from scratch, and then export the results into a PowerPoint (or some other office document). Often, stakeholders would request revisions, resulting in more ad hoc programming, emailing back-and-forth, and so on. This process could take weeks or sometimes even months!
The result is what we call the “data-to-knowledge bottleneck”. This bottleneck leads to a vicious cycle: The data science team can’t process requests quickly enough. So eventually stakeholders stop submitting so many requests. Now the data science team can keep up with the requests, but only a tiny, tiny fraction of the data is being used to generate any real value for the organization.
To break through the bottleneck, our client tried standing up “living” data dashboards (with tools like R Shiny or Plotly). Unfortunately, those didn’t satisfy their stakeholders. This is because data is not the same thing as knowledge. Knowledge requires context and interpretation. Knowledge requires a narrative.
This is part of why a scientific paper isn’t just the methods and results section. To know why the methods and results are important in a paper, we need the context of a specific hypothesis. With data dashboards, you might get a ton of cool-looking charts, but if you don’t have the narrative to tie them all together, you still don’t have knowledge. As our client said, “Everyone does R Shiny dashboards these days. We've got 100s of them in different states of abandonment.”
In other words: With a dashboard, you are still asking the stakeholder/audience to do a lot of the work themselves. They have all these displays and nobs and filters to play with, but what they want is a clear presentation that answers their questions. What they want is a narrative supported by credibly data and rigorous analysis.
This is exactly what Prism.bio’s platform–or more specifically, what our Prism Writer product–provides. From custom R&D visualizations to sophisticated annotations written by our AI agents, our platform made it possible to go from raw data to publication-quality report in a minutes. With Prism Writer, our client finally broke through the data-to-knowledge bottleneck. Their data science team was able to produce rigorous reports with a new level of speed and polish. They were satisfying stakeholder requests. They were finally reversing the vicious cylce and seeing the demand for reports increasing.
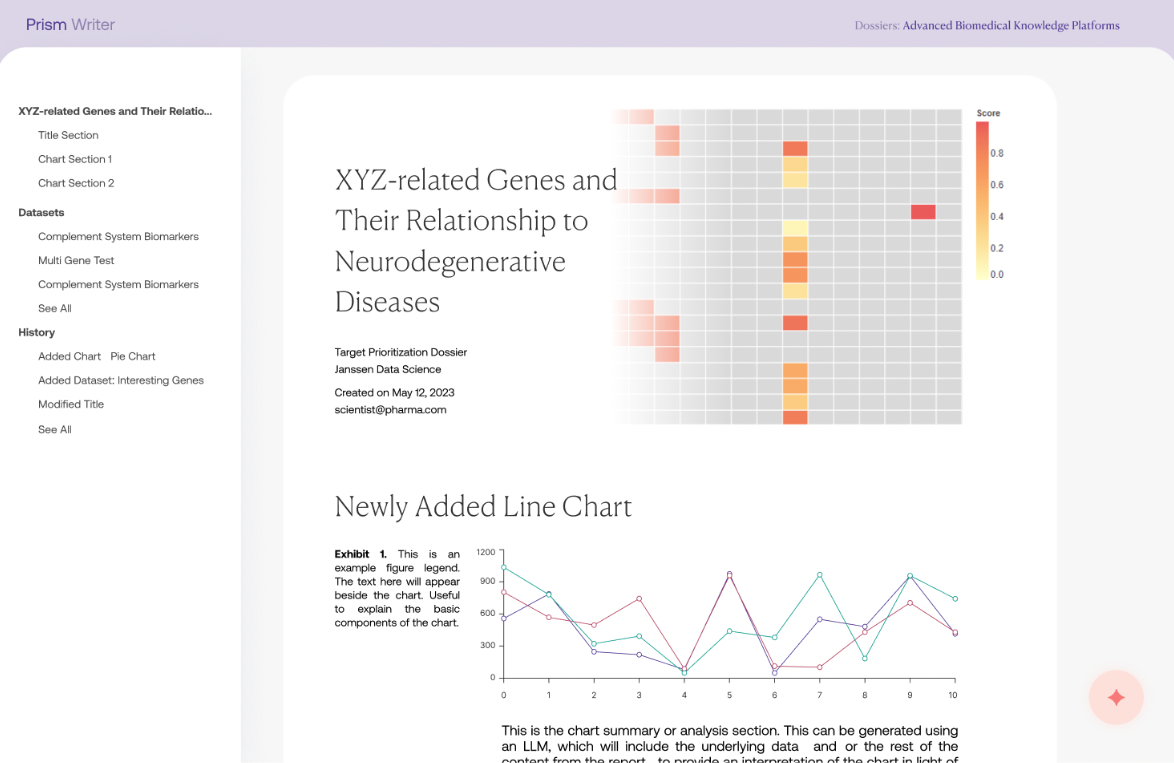
The bottom line: Prism Writer’s reports get the job done. Internal stakeholders love them because they are polished, engaging, and easy to read.
They are also easy to share. Prism Writer reports are now a focus in strategy meetings with the heads of therapeutic areas, and they are getting featured at internal science expositions.
With Prism Writer, our client’s data scientists are now immeasurably more efficient. As a result, the vast data at our client’s organization is finally being put to good use–guiding them toward better, faster decisions on target selection, prioritization, drug safety, and more. These are all critical steps in the early drug discovery and development process, which means that the efficiency gains are not limited to just data science, but accrue to R&D as a whole.
Interested in exploring what the platform could mean for your company? Request a demo today.
Latest Articles
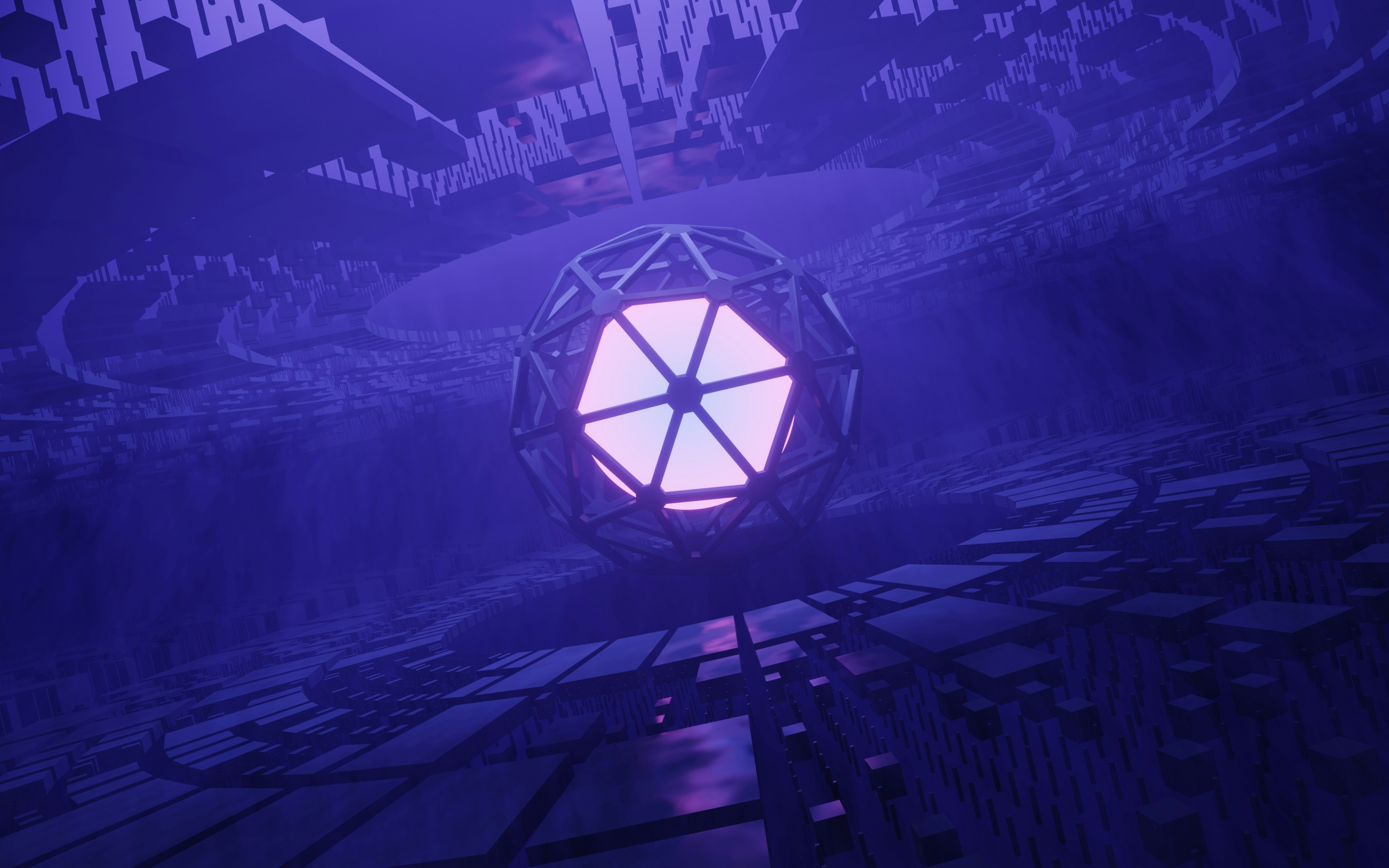
Understanding Large Perturbation Models
A brief, layperson's introduction to Large Perturbation Models (LPMs), a new tool in the drug development toolkit to simulate vast numbers of experiments digitally