How evidence landscapes contribute to the success of precision medicine
Written By:

Predictive biomarkers are measurable characteristics of a patient that can tell us something about how a patient is likely to respond to a particular course of treatment. Such biomarkers are fundamental to the success of Precision Medicine—a paradigm of biomedicine that tests patients for predictive biomarkers in order to better inform treatment decisions. When proven effective, precision medicines are truly amazing: Patients with a specific biomarker get a precise, effective treatment, while patients without are spared unnecessary toxicity and burden. However, clinical trials for precision medicines are inherently more complicated than traditional clinical trials, and if not planned carefully, their results can be uninformative.
For example, if a precision medicine trial doesn’t show that a drug is beneficial, that doesn’t necessarily mean that the drug is ineffective. Perhaps the drug does work, but there was something wrong with the test used to measure the biomarker. Or perhaps there was something wrong with how the patient specimens used for biomarker testing were collected. Or perhaps the drug is beneficial for patients that have a different biomarker altogether!
Even if the trial seems to show that the precision medicine is effective, if the trial is not designed in the right way, we still might not know if the biomarker is giving us useful information. It could be that the treatment is just better for everyone and the biomarker is giving us bad or useless information.
In the philosophy of science, this challenge for interpreting the results of complex experiments is called “The Problem of Underdetermination”. Every experiment supports multiple interpretations, and so making wise decisions about R&D will require a strategy for how to interpret experiments and inform next steps in a product’s lifecycle.
In this publication in Science Translational Medicine, Prism’s co-founder, Spencer Hey, and his academic colleagues, argue that step 1 for developing such a strategy is mapping out the parameters that need to be tested in order to know how to use a precision medicine. The basic scientific insight here is that if you don’t have a working, up-to-date picture of the evidence landscape, then you don’t really know what has been done, you don’t know what you don’t know, and you don’t know where you are going. So how can you possibly make wise decisions about future research?
The strategic power of evidence landscaping is one of the cornerstones of Prism’s philosophy. Our platform makes it fast and easy to construct an evidence landscape—even for scientific domains that are as complicated as precision medicine.
References:
Hey SP, Gerlach CV, Dunlap G, Prasad V, Kesselheim AS. The evidence landscape in precision medicine. Science Translational Medicine. 2020;12(540):eaaw7745. https://doi.org/10.1126/scitranslmed.aaw7745
Hey SP, Kesselheim AS. Countering imprecision in precision medicine. Science. 2016 Jul 29;353(6298):448-9. https://doi.org/10.1126/science.aaf5101
Latest Articles
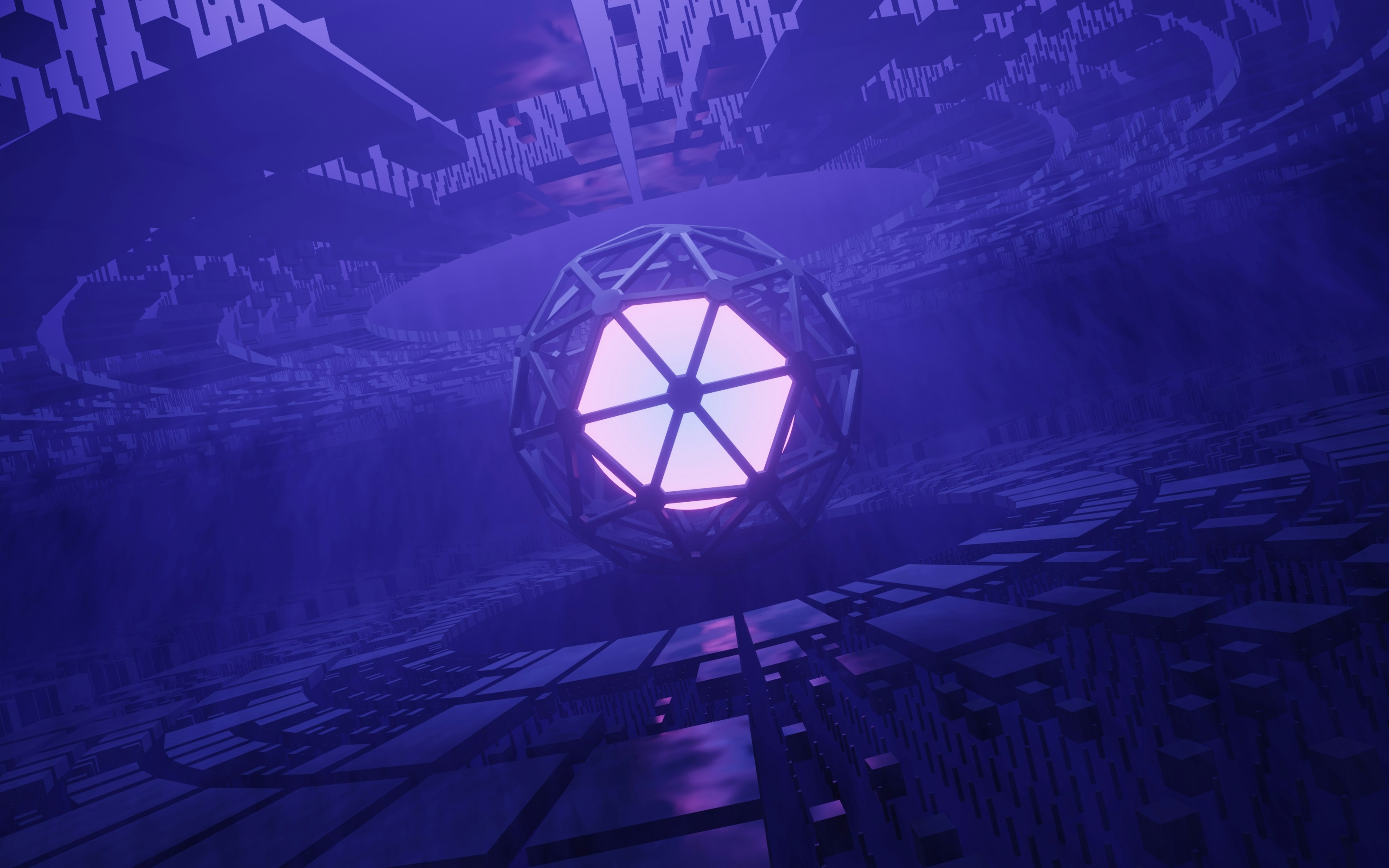
Understanding Large Perturbation Models
A brief, layperson's introduction to Large Perturbation Models (LPMs), a new tool in the drug development toolkit to simulate vast numbers of experiments digitally