Heuristics and scientific judgment
Written By:
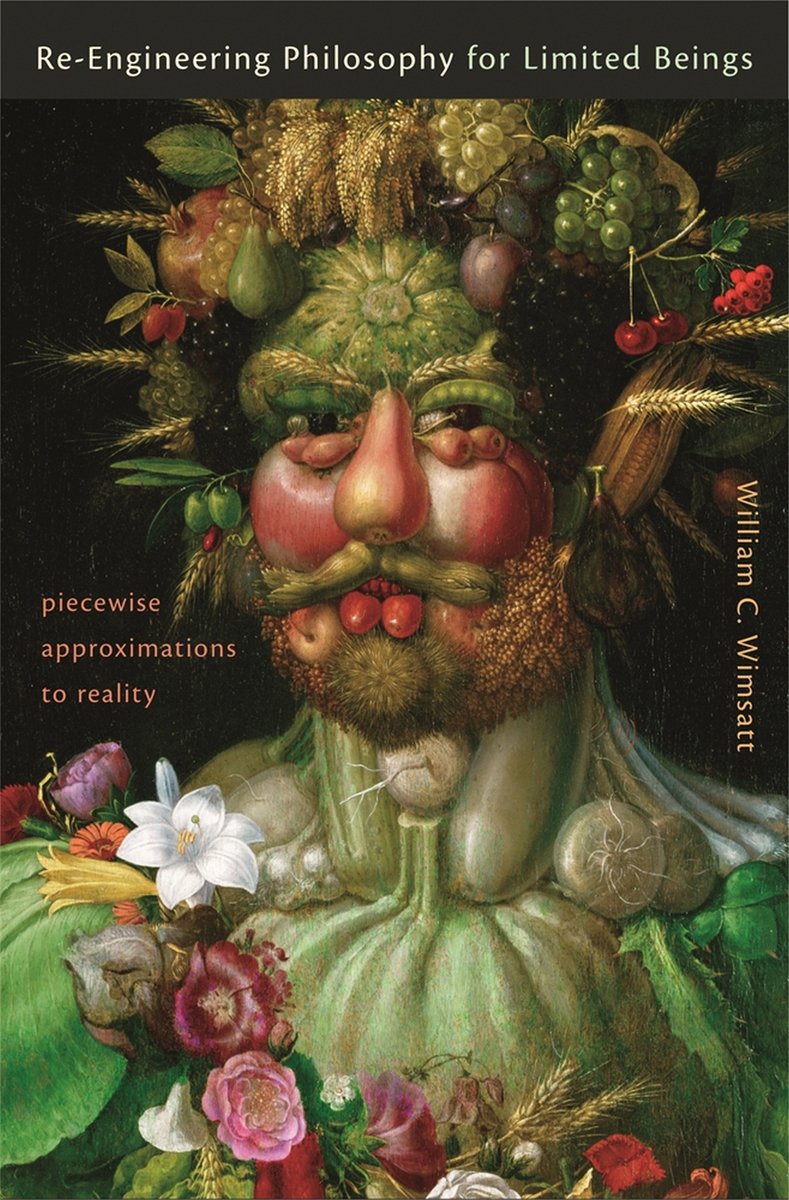
In the previous post in this series, I wrote about how Prism’s “intellectual life” began out of a long-standing challenge in the philosophy of science—namely, how to characterize what is “good sense” in the context of scientific judgment.
How does a scientist decide what questions to ask? How do they determine the best methods to answer those questions? How should they combine and weight different bodies of evidence? How should they test and revise theories? My PhD thesis offered a theory of what makes “good sense” for scientific judgments like these. For that work, I drew heavily on the ideas of Bill Wimsatt.
In this post, I will introduce some of Wimsatt’s ideas and describe how they influence what Prism is doing today.
In his book, “Re-engineering Philosophy for Limited Beings: Piecewise Approximations to Reality,” Wimsatt develops a compelling account of scientific heuristics, building on ideas from cognitive psychology and artificial intelligence, from thinkers like Herbert Simon and Douglas Lenat. He defines scientific heuristics as cost-effective, albeit biased, procedures that can help us “limited beings” (i.e., humans) simplify and solve problems.
He then lists the myriad ways in which scientists rely on heuristics when they are testing theories, designing experiments, and interpreting evidence.
And this wouldn’t be a proper story involving Wimsatt, if it didn’t also include at least an excerpt of one of his lists (note: Wimsatt’s work is delightfully full of lists).
For example, his “heuristics of model building and theory construction” include (excerpted from this publication):
- Modelling localization: look for an intra-systemic mechanism to explain a systemic property rather than an inter-systemic one
- Contextual simplification: simplify the description of the environment before simplifying the description of the system
- Generalization: when improving a simple model of a system in relation to its environment, focus on generalizing or elaborating the internal system structure, at the cost of ignoring generalizations or elaborations of the environmental structure
The basic idea with each of these heuristics is to abstractly describe effective strategies for simplifying, explaining, or theorizing. Applying these heuristics will reduce the complexity of the problem under study at the cost of ignoring some information and thereby introducing a bias.
But although Wimsatt is careful to emphasize the biases that can result from their use, his take-away message is not to avoid using heuristics. Indeed, that would actually be impossible! Science requires heuristics.
The take-away is rather to understand and be aware of the biases that each heuristic introduces, and then recognize if/when these biases are going to cause a problem. The wrong heuristic can lead you to the wrong answer for the problem you are trying to solve. The wrong heuristic can also conceal important questions or truths.
But the right heuristics for the right situation is what gives science its power. By simplifying and throwing away some of the information, science can reveal the patterns, mechanisms, and laws that characterize our universe.
Therefore, to wield this power wisely—i.e., to do good science—will require a conscious understanding of the relationship between the scientific problem-to-be-solved and the heuristics involved in its possible solutions.
And thus are we led back to the challenge of “good sense”. If scientific judgments can be understood as heuristics—which are defined as reliable-yet-imperfect rules for designing experiments, interpreting evidence, formulating hypotheses, etc.—then “good sense” can be understood as meta-heuristics—which we can define as reliable-yet-imperfect rules that govern the wise use of scientific heuristics.
For my thesis (and in several other publications), I went on to show how this kind of meta-heuristic model for understanding “good sense” and strategic scientific judgments opens up a powerful framework for analyzing scientific R&D programs. In essence, once we can appreciate how individual scientific experiments are guided by heuristics, a meta-heuristic approach provides a conceptual framework for studying the higher-level heuristics that guide the overall R&D program.
This framework allows the scientist to better diagnose errors and predict outcomes. It allows them to systematically study and improve their strategic thinking. It is the epistemic foundation for everything we do at Prism.
In the next post, I will describe the first application of the meta-heuristic framework to a real-world R&D program. In collaboration with researchers at the U.S. Centers for Disease Control, I used this framework to evaluate a “go/no-go” decision for an antibiotic drug development program, and the result was an entirely new method for visualizing and analyzing data in clinical research.
Latest Articles
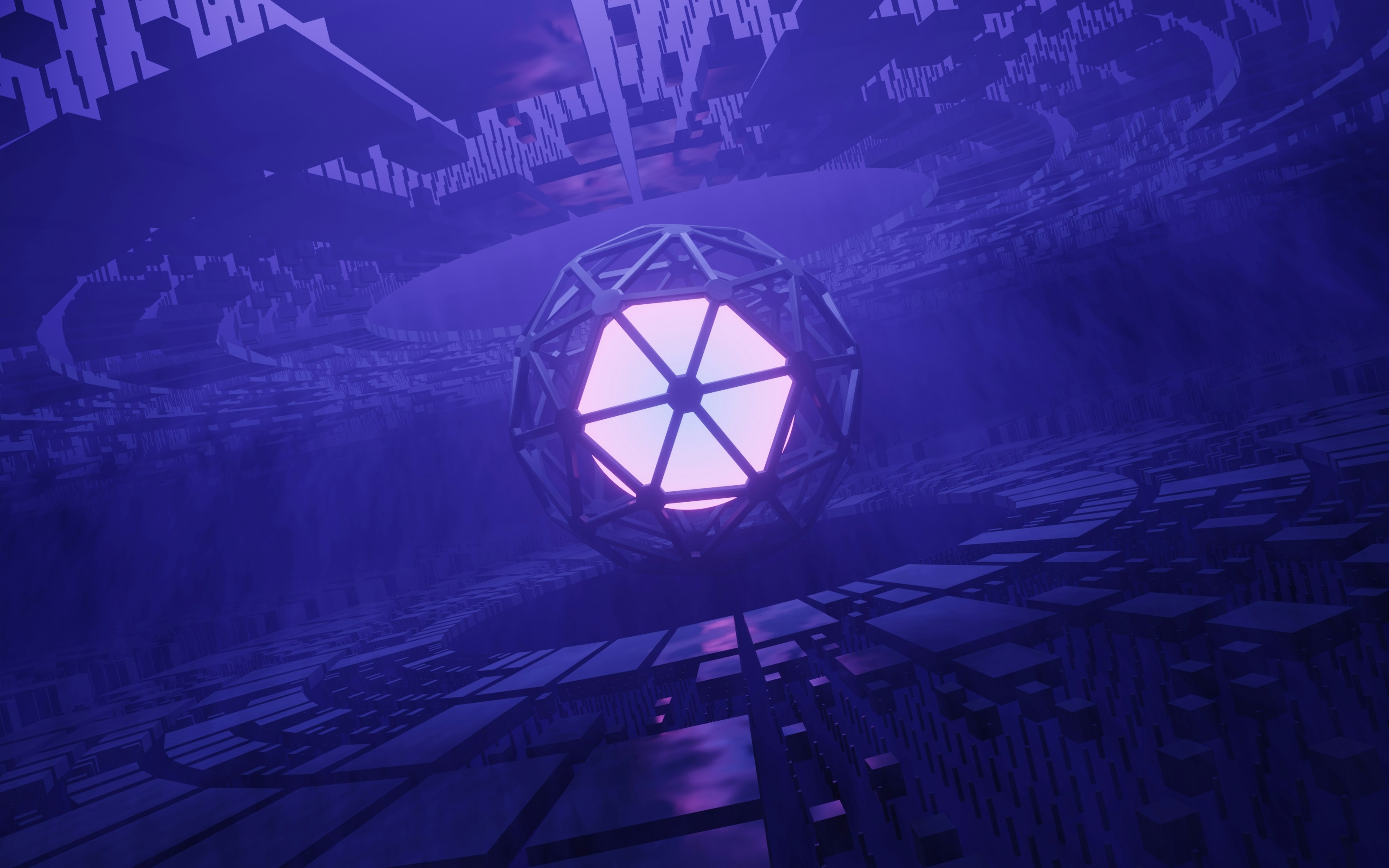
Understanding Large Perturbation Models
A brief, layperson's introduction to Large Perturbation Models (LPMs), a new tool in the drug development toolkit to simulate vast numbers of experiments digitally