From Data Void to Data Driven: Mapping the Landscape of Digital Health Technologies
Written By:
Spencer Hey
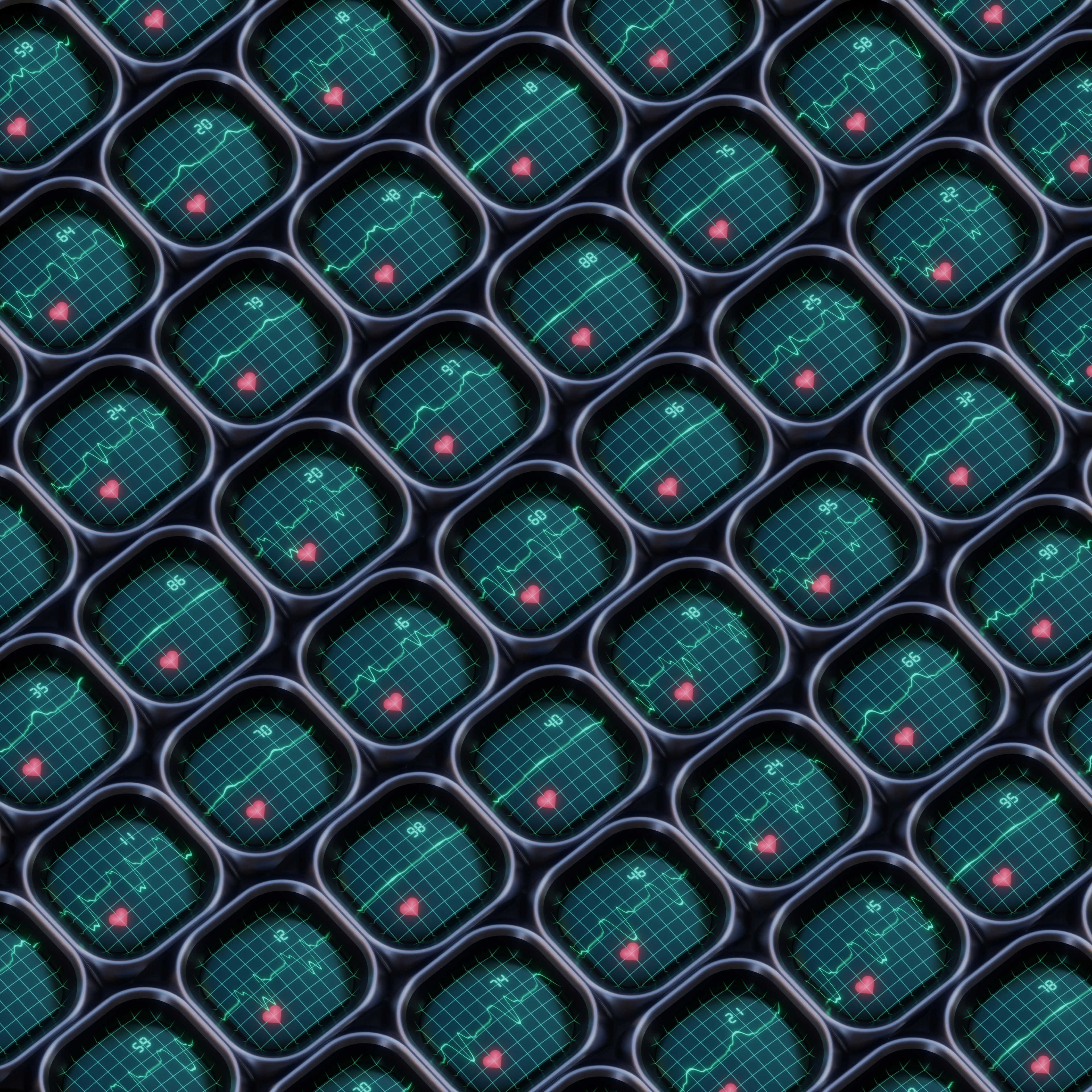
It is no secret that digital health technologies (DHTs) are revolutionizing healthcare. From telehealth apps to image recognition AI to smart packaging, there are numerous ways that DHTs can enhance the quality of care.
However, as other commentators have observed, the marketplace for DHTs is a rapidly growing frontier with significant risks and uncertainties—a place where regulations can struggle to keep up. For healthcare providers, navigating this marketplace to evaluate the supporting evidence for specific technologies can be challenging.
This challenge (I would argue) is even more acute when it comes to DHTs used as instruments in clinical trials.
DHTs undeniably offer a wide range of potential benefits for conducting clinical trials. They have the potential to alleviate the burdens on research participants by enabling outcome monitoring without the need for clinic visits. They could expand participation to a more diverse population, including those who live far from major research centers. When a DHT has undergone rigorous validation as a measurement tool, it can improve the quality of data generated in a clinical trial, thereby enhancing the study's scientific value.
Unfortunately, the scientific ecosystem for DHTs in clinical trials is fragmented and full of so many gaps that it can fairly be described as a “data void”.
Trial registration platforms, such as ClinicalTrials.gov, do not require sponsors to report their use of DHTs. Journals do not require authors to provide detailed, structured information on DHTs in the published study results.
I know from speaking with several DHT manufacturers that some do not even track the information about if, how, or how many of their devices have been used in trials.
This lack of standardized data collection and reporting makes it almost impossible for the scientific community to know which DHTs have been used in trials, how they were utilized, and their reliability as measurement instruments. And without some kind of structure or standardized method for reporting, this situation cannot improve. Even if all trial sponsors and DHT manufacturers suddenly agreed to share all their data on DHT use, there would likely be so much heterogeneity that a valid analysis would be impossible.
This is another facet of the data void—a lack of scientific visibility because of siloed, fractured, partial, non-standardized, or otherwise low-quality data. In the case of DHTs, this is no particular stakeholder’s fault. It is also no particular stakeholder’s responsibility.
However, I believe we (i.e., the scientific community) can do a better job of filling in this data void. Here is how:
Last year, Prism.bio collaborated with Janssen to develop and publish the world's first scientific ontology for DHTs. This is now published on Stanford's BioPortal repository for ontologies. It is available for anyone to use or improve.
This immediately helps address one of the causes of the data void by standardizing the collection and reporting of data on DHT use. If there is a common ontology, stakeholders to the DHT ecosystem now have a framework to record and share interoperable data on DHT use.
But we didn’t stop there.
Using this ontology, we conducted a systematic analysis of devices reported in ClinicalTrials.gov. Even though registration platforms do not mandate sponsors to report DHT use, some sponsors (thankfully!) do voluntarily provide this information (albeit in a largely unstructured form). We created an algorithm to automatically search the registration records for mentions of specific devices and then structure this data according to the ontology. Then we analyzed the results and published our findings.
What did we find? The histogram below shows the number of trials reporting DHTs from the ontology by trial start date, stratified by the type of trial sponsor. This shows steady growth in the uptake of DHTs in trials over the past 20 years, with a noticeable jump in 2015. It also shows that universities have been the largest drivers of this activity, followed by research hospitals. Industry, by contrast, has been relatively slow to adopt (or at least report on adopting) DHTs.
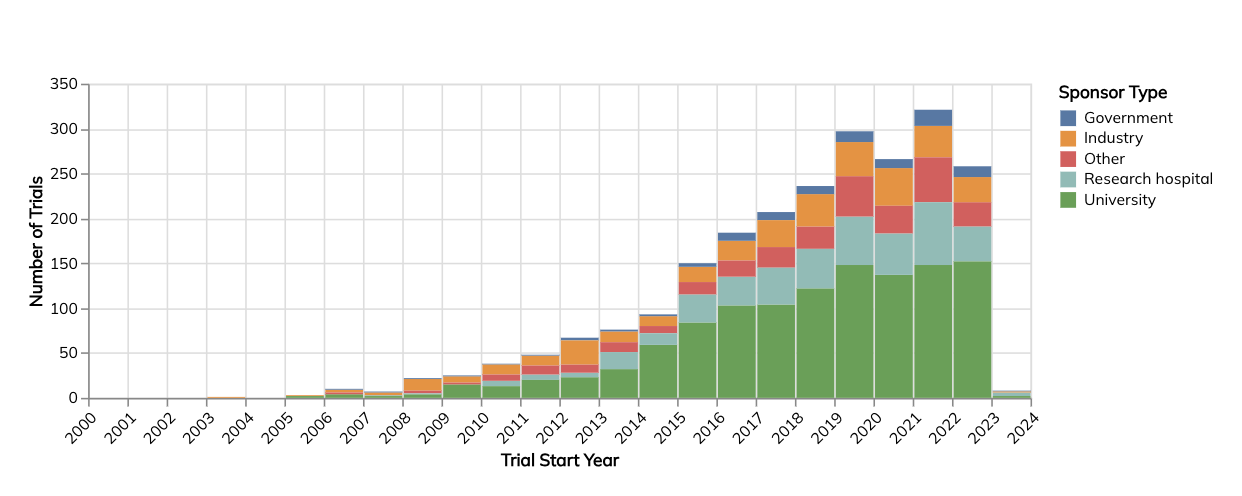
The chart below shows the top 10 most-studied disease areas in these trials, based off of the MeSH terms in the ClinicalTrials.gov record. The most frequently studied area was Pathological Conditions, Signs, and Symptoms, but this is not all that informative since so many MeSH terms are grouped under this heading. However, the rest of the categories are more informative. The activity in Nutritional and Metabolic Diseases and Endocrine System Diseases is driven by diabetes research, which makes sense given the update of continuous glucose monitor devices. The activity in Nervous System Diseases also makes sense, given that many wearable DHTs measure actigraphy, useful for evaluating movement disorders. Similarly for Cardiovascular Diseases—since many DHTs include heart rate monitors.
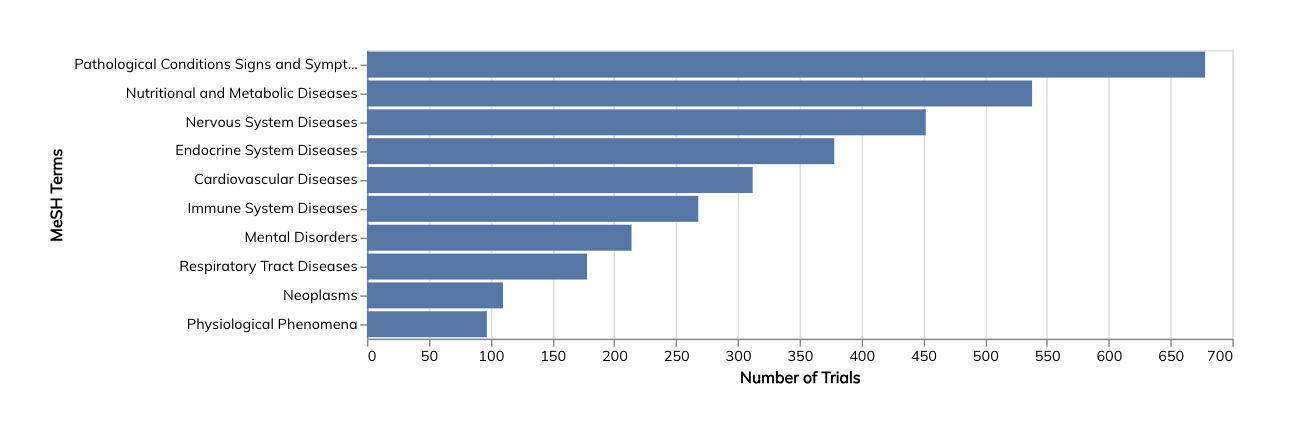
But these high-level summaries are just the tip of the iceberg. There are many more insights to extract from the data. To help others to find these insights, we made all the data available to download as a part of the supplementary materials with our publication.
However, I’ve always disliked the scientific practice of saying, “If you have more questions about my data, you can go and analyze it yourself.” It’s like the equivalent of “throwing work over the wall”.
So we didn’t stop there.
We’ve now created a public "knowledge vault" that more thoroughly illuminates all this data, analyzing and summarizing it for faster, easier consumption.
In this vault, every device, device class, manufacturer, and measurement component has its own dedicated analysis. For example, let’s say you want to know more about trials involving just wearable devices. That analysis is here. Since wearables make up the largest portion of devices in the ontology, the results for this subset of DHTs closely mirrors that of the total dataset. In the chart below, we break down the trials by phase, and it clearly shows that phased trials (which are typically drug trials) make up a tiny portion of the DHT trial landscape.
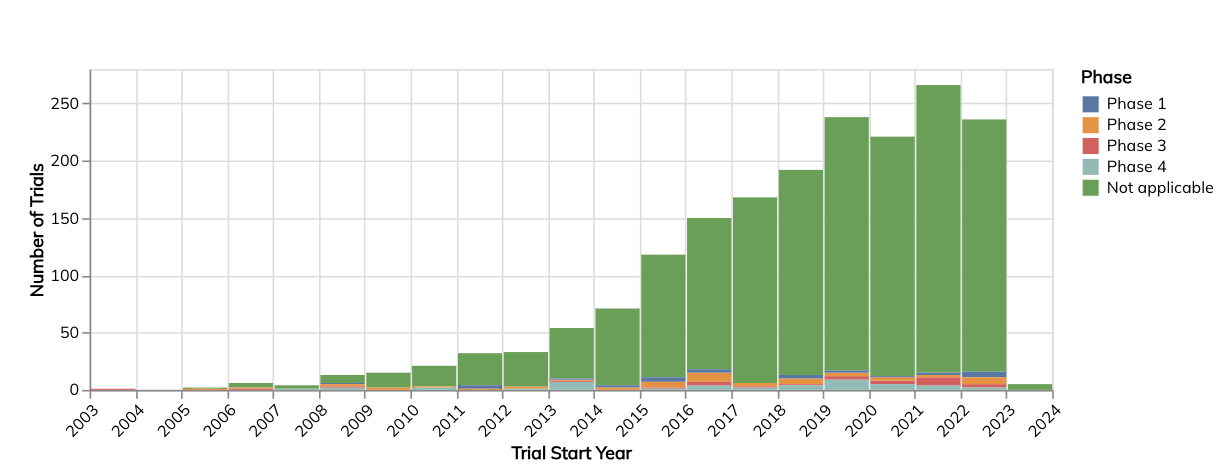
But we can drill in even further. What about trials with smartwatches? That analysis is here. The chart below shows the top-10 most used smartwatch devices from our dataset. The Polar RS800CX was the most used device, followed closely by FitBit’s Charge 2. So if I am planning a trial and contemplating including a smartwatch, this list gives me a good, data-driven starting place.
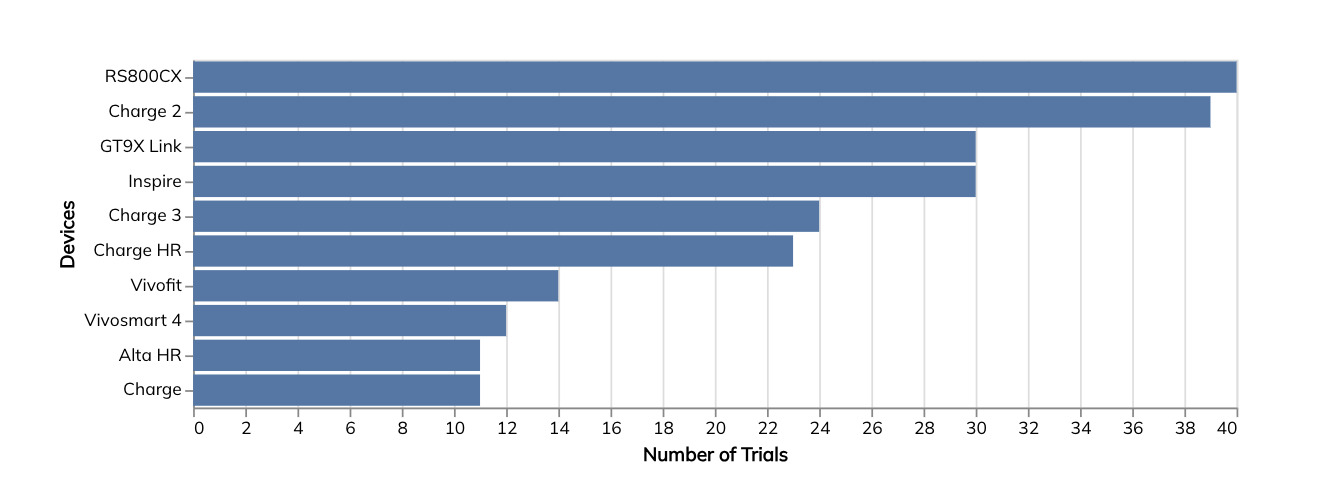
Now let’s say I like the idea of using a FitBit device, but I know that the Charge 2 is a bit of an older model. So I’d like to explore what other FitBit devices have been used. The analysis of all FitBit’s devices is here. It shows me that the Flex, Inspire, Zip, and many more FitBit devices have also been used frequently (e.g., more than 20 trials each). It shows me (with the chart below) the top-10 sponsors that have most often conducted trials with FitBit devices. The top sponsors are all academic institutions and research hospitals, but if I am looking for signs of reliability, the fact that many top-tier research institutions have used FitBit DHTs in multiple trials would indicate that these devices have earned trust. I would take that as a good sign.
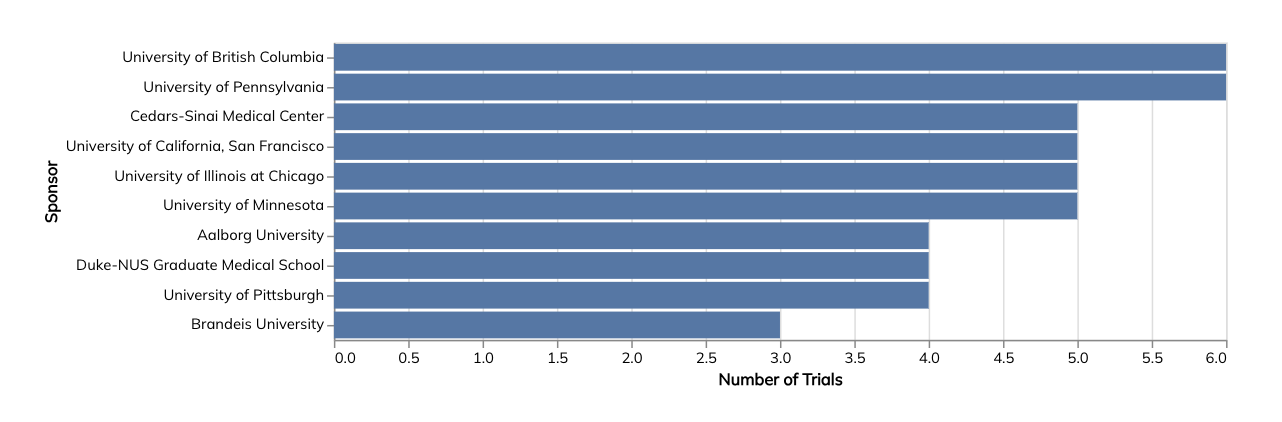
All of these analyses and more are available in the knowledge vault. The goal of the vault is to analyze, organize, and share all the data that we generated on DHTs in trials, not just that highest-level fraction that can fit within the limitations of publication. I’ve just suggested one workflow that someone could use to identify a potential smartwatch suitable for use in a trial (i.e., drilling down from wearables into smartwatches into FitBit). But there are thousands more paths through the vault that can help support decision-making.
That said—this vault is certainly only a first step to a more complete, data-driven understanding of the domain. To truly transform our understanding of DHTs in trials, the vault, the data, and the ontology underlying it will all need to grow and evolve. In that spirit, I welcome all feedback.
I know that in its current state, this vault is still only capturing a fraction of the actual DHT use in trials. I also know that there are many more questions that trialists contemplating a DHT would need answered. But despite those limitations, there is nevertheless a powerful a vision of the future here. This is a future where the data on DHT use in trials is tracked and shared in an accessible format; where the analysis and perspective needed to intelligently fold DHTs into your clinical trial is ready whenever you need it.
Latest Articles
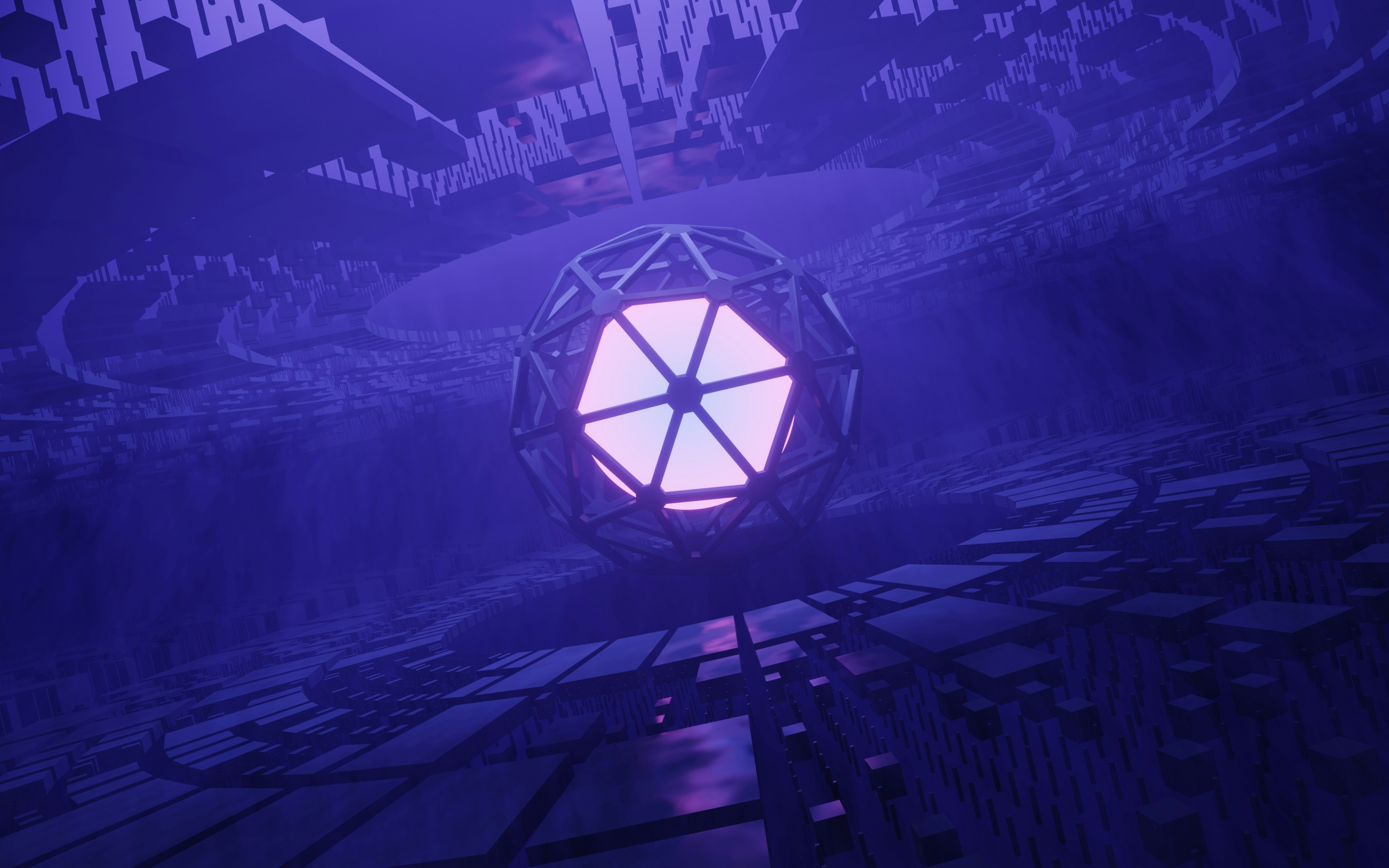
Understanding Large Perturbation Models
A brief, layperson's introduction to Large Perturbation Models (LPMs), a new tool in the drug development toolkit to simulate vast numbers of experiments digitally