Discussing Software as a Medical Device with Shrawan Patel
Written By:
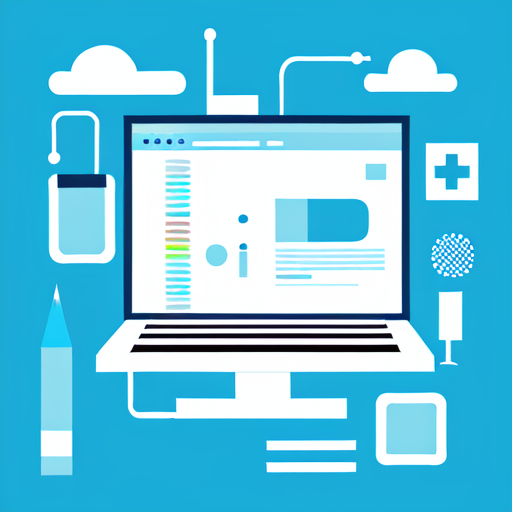
In this month's "Discussing Data Science" episode, I talk with Shrawan Patel, managing director at Strategy Health and a founding member of PharmStars. You can watch the video below or on Youtube. But if you'd prefer to read, keep scrolling. The complete transcript (edited for length and clarity) is below.
Transcript
Spencer Hey (SH): Hello, my name is Spencer Hey. I am the co-founder and chief science officer at Prism Bio, and welcome to “Discussing Data Science”. My guest today is Shrawan Patel.
Shrawan has long been working at the forefront of digital medicine. He previously worked as a physician for the NHS in London before moving to Mount Sinai in New York to become the clinical transformation lead for the Digital Innovation Center. He's also been an angel investor and startup advisor. He's owned and operated several businesses, and the list really goes on.
Shrawan is currently managing director at Strategy Health, a boutique consulting firm helping those in the healthcare space to make the most of new digital health technologies. He's also a founding team member and mentorship director for PharmStars, the pharma-focused accelerator for digital health startups. So, Shrawan, welcome to the show and thank you so much for joining me today.
Shrawan Patel (SP): Thanks for having me, Spencer.
SH: So why don't we start with a little bit about your background. You have a rich backstory here of how you came into the digital health space. I'd love to hear you say a little more about your journey.
SP: I think my journey has been definitely a bit fortuitous, and certainly not straight by any means. Prior to med school, I started and ran a number of businesses. When I started at med school, what I had been doing academically and what I had done outside of school had always been very separate. I thought med school might be the time to try and bring those things together. I ended up working with research labs and spinning tech out. Now, my alma mater, Imperial, has a very good tech transfer office and commercialization office. We're talking about 15 odd years ago, and I think things were still pretty early, and so there wasn't a good route for research to get out of the lab into the wide world and actually function in a commercial manner. I worked with a number of research groups in setting up companies, securing early-stage funding, building business plans, etc.
My friends from med school, many of whom weren't medics but engineers, chemists, mathematicians, suggested I learn Python, an up-and-coming coding language then. With their advice, I started learning Python, which eventually led me to learn JavaScript, HTML, CSS, and I managed to develop full-stack developer skills.
Being in London around 2007-2008, during the early stages of digital health, and having some business experience, it made sense for me to get involved in health tech companies. I continued this work in London even after I started practicing. When I moved to the United States about seven years ago, I joined a large health system and combined the clinical knowledge that I built up working as a physician with the tech piece. That's when I started working at Sinai with the Innovation team and thinking about how digital health solutions are being used across our system.
My first foray into working with pharma started when I began working for a startup that had spun out of the health system. We had a pharma company reach out saying they'd heard we were building something interesting and would love to learn more. Navigating those initial conversations and the contracting process was a bit of a stumble, but we managed to get it across the line. We ended up having a number of deals with pharma companies, which is a lot of what I bring to PharmStars.
About three and a half years ago, around the time my son was born, I left Sinai and the startup and started working with other health tech firms. That's how I started Strategy Health. Over time, as clients and projects have grown, we've found our niche working with Software as a Medical Device (SaMD) companies. We work across the whole spectrum of SaMD, from designing protocols and running clinical trials, to working with clinical teams, product teams, data science teams in building SaMD FDA applications, and then doing commercial work afterwards.
At PharmStars, we work with digital health companies, helping them understand the pharma space so they can position their products in a way that will be of interest to pharma. We then put them in front of the pharmaceutical companies that are members of PharmStars, such as large companies like Eli Lilly, mid-sized companies like Nova Nordisk, and smaller firms like Alexion. The idea is that if you remove some of the distance between them, deals are more likely to happen.
So that's the path from med school through to where I am now and what I do.
SH: That is awesome! As you say, maybe not a straight line, but I don't know that anyone takes a straight line, to be honest. You know, there's a saying that if you're innovative, your path isn't going to be straight; you're going to veer.
SP: It's true. I think there are lots of straight lines to get places, but I think there are certain types of people that maybe decide to take an alternative route.
SH: Perhaps from a biology, medicine, or engineering background there are some straight paths into pharma. But if you're kind of searching around for what's new, and where are the big opportunities to really do things differently are, you're going to wind a little bit, right?
Trials for Software as a Medical Device
SH: I want to delve into the clinical trial experience a bit. I'm keen to hear more about the trials and tribulations of software as a medical device - conducting trials and evaluating software for regulatory approval. It's exciting. We've had guests on the podcast already who have experience with this, but it seems like this domain is still somewhat figuring out how it's going to work exactly. I'd love to hear more about your experience there.
SP: There are many open questions when it comes to software as a medical device. Some of the big questions that plagued the industry three or four years ago have begun to close. Things like reimbursement pathways are becoming a bit clearer. It doesn't mean the issues have been resolved or answers found, but the potential solutions are becoming clearer.
What I've noticed when we start working with companies is a contradiction with software as a medical device, especially for small startups. If you're a small company building something classified as a software as a medical device, you're still pulling funding from investors who primarily invest in tech. They will see you as a tech company because you're building software. But the way a tech company operates and the way a medical device company operates are vastly different.
So, there's a contradiction where expectations are one thing, and reality is something else. People building these medical device software products often don't think about compliance, regulatory, and commercial aspects until later down the line. It's easy to present a slide showing a huge market opportunity, say, a tool that could potentially diagnose asthma. The potential market is huge, but how do you get paid for your product?
Market sizing is very different from business models. Business models may change, which is fine, but you need some clarity that a business funnel exists. For many software as a medical device companies, the business model may not exist. It could be because healthcare is a bit unusual in how it operates. For instance, there's a product I know that can diagnose a sleep disorder. It's a fantastic tool, but if it gets used, it cuts a significant revenue source for most hospitals - the diagnosis. So a clinically excellent product may be detrimental to the financials of the health systems you're trying to sell into. This is just one example of a situation where the business model doesn't support what should exist, almost to the point of non-existence.
Regulatory and compliance are often left until later down the line, and that's a problem with trials. Commercial aspects are also left behind, but these things need to be considered when you start your protocol development process and design your trial. Every data scientist knows that the data you collect in your study needs to be the right data to produce the product you want to build. You can't go back to the patients in your study retrospectively and ask them a question you've realized is crucial for the algorithm. Your data, in many ways, defines the type of product you can build. Once you start building your product and train your algorithm to give certain outputs, the labels and claims on your product, and where the product gets used, are all affected.
From a regulatory standpoint, if you've collected your data in one setting but want to use the product in a different setting, the regulator might say you have no usability data showing that your algorithm can be applied in a different place. These knock-on effects of not collecting data correctly mean the product doesn't function properly, which means what you hoped the product would be can't happen. It all starts from the beginning, because if there are any mistakes or issues along the way, you have to go back and redo things since you're working in a regulated environment.
SH: That's fascinating. If you think about the startup wanting to develop software as a medical device: The received wisdom is "build an MVP, then iterate, iterate, iterate, don't wait to put something out there." It sounds like there's a fundamental tension there, too, right? Before you can even get going, you have to know what you're gonna do. How do you solve that?
SP: I'm not sure that there are ways, or obvious ways, in which you can change that because the stepwise process, the way regulations and compliance is written, is there for a reason. And I think actually the reasoning behind a lot of these things is good and there should be some accountability. But those also mean that doing iterative changes and moving fast and breaking things is also not really that easy to do. But I think there are, there is opportunity for, I guess, changing the approach a little bit.
So one of the things that we do with startups is help them realize and build studies that are actually really low cost and low burden. I think people think of clinical trials and they think of the $5, 10, 20 million pharma trials. But what they don't realize is that they could go to any clinician. Clinicians don't even need to be PIs. The PI just needs to be someone that is appropriate to run the study or to head the study. So you need someone that can act and function as your PI. It doesn't really matter that much who that person is, you don't need the top surgeon at Mayo Clinic to be your PI. You just need to find, if you're building something for the surgical space, you just need to find a surgeon that would be willing to do it.
And then you build your protocol, you go through your IRB process that's going to be in the institution or if it's a decentralized trial, you have a central IRB. I think people don't realize that. I think doing a protocol submission through some of the most common IRBs is going to be, you know, a few thousand dollars. You'll have some extra bits of documentation that are going to cost a couple of hundred bucks each, but overall to get your trial through ethics is going to cost let's say six, seven grand. Plus, your PI, all the tech, and all the build is on your end.
There are now companies, these electronic data capture (EDC) companies, that can actually do pretty good things at a low cost. So running and putting together a study doesn't have to be a million-dollar affair. You can run small-scale studies, and you can pull basic data. And then you get into the data science principles. Because you can do things like cross-validation, right? So you can both train and test on the same dataset.
So that's not really medical device-specific, that's data science-specific, but you can take a relatively small dataset and have a pretty good finger-in-the-air guess as to, "Yeah, we think there's something there." You can then open that data, have it as an open-label study, look at the data as it's coming in, and then do the "move fast and break things" data science process, right? So you're doing your algorithm development in that fashion as you'd want to, but it's in a trial database, in a trial setting.
But you're also doing that knowing that it's deliberately small and you're going to have to do a bigger version. But now you have enough data to be confident that you can do a larger pilot study to collect more data.
You then build that study based on what you know needs to be the case for your final product. And then you might build in another arm that is made up of a blinded dataset that you can then do a prospective assessment on. It's like there are clever ways of doing it, but I think it's a set of skills and a knowledge base that's still building up. Fast forward five years, ten years, and I think things like that or that way of thinking will be a lot more common. And then the world's the oyster.
Are the Regulatory Agencies Ready?
SH: Do you think that the regulators are prepared to effectively handle the changes that you see happening? In particular, do you believe they are prepared for a potential surge in volume of software as a medical device products?
SP: Well, I recently read a headline that suggested the FDA might not be fully prepared yet. But isn't it the role of regulators, not just in healthcare, but in every sector, to be in a constant state of catch-up? This is no different for the FDA. They're bound to lag behind innovation a bit, and there's nothing inherently wrong with that. It's simply how the world operates.
Over the past few years, the FDA has shown remarkable progress in their openness to innovation, building digital Centers of Excellence, trialing initiatives like the pre-certification program, and continuously releasing and improving guidelines focused on AI and software as a medical device. From what I've observed, the communication with regulators through pre-submissions has notably improved.
I commend the FDA for their proactive approach. They understand the necessity of being part of the conversation and have shown this through their dialogues with startups and industry organizations. Their aim is to understand the direction of technological advances and consequently, to establish rules and regulations that balance safety and innovation.
Many people forget that the primary role of the FDA is to ensure safety. Efficacy is essential, yes, but safety is paramount. When engaging with the FDA, companies aren't required to constantly prove they have the best product in terms of efficacy. However, they must always demonstrate that their product is safe.
The FDA currently grapples with complex questions. For instance, should all algorithms be locked before FDA approval and subsequent market launch? If they are, how does this affect product improvement and development? Is it feasible to allow algorithms to self-learn and adapt, and if so, how can safety be maintained? The pre-certification program was designed to address some of these issues. It aims to pre-certify companies, giving them a little more freedom to go through product development cycles and release products.
The focus might shift more towards real-world evidence generation and requirements. Similar to drug regulations, this would allow a product to launch on the market with certain conditions. The product could evolve, but companies would have to continuously prove its safety to the FDA.
SH: It sounds like you're describing a form of accelerated approval process.
SP: Yes, exactly. And though I certainly don't envy the FDA's position, I commend them for their open-mindedness and dedication. From what I've seen, they are taking the right steps.
What are the biggest data gaps in the field?
SH: Let's transition to our standard three questions that we ask every guest. The first one being: "What do you perceive as the most significant data gaps in the field?" Where are you finding yourself saying, "If only we could bridge this gap?"
SP: Because of my experiences in different sectors, I think the answer varies depending on the perspective.
From a startup's perspective, especially those developing software as medical devices, one of the most glaring data gaps is the availability of open-access databases. If a startup has a promising idea and wants to test its viability, their options are limited. They either have to undertake a costly and time-consuming data collection process or conduct a study. However, organizations like Sage Biosciences are doing commendable work by creating open-access datasets that startups can use to assess their ideas and discover novel product opportunities. The number of such databases is increasing, but for startups, this gap remains significant.
As for the pharmaceutical industry, there are numerous data gaps. An interesting point was brought up by a company in the last cohort of PharmStars: They focused on the "capability gap" in answering medium complexity and medium value questions. The business model of pharmaceutical companies revolves around posing questions, conducting studies to find answers, and then developing drugs based on those findings. For high-level, complex questions, multi-million-dollar clinical studies are justified. Lower-level questions can often be answered effectively using registries or real-world evidence. But what about those mid-level questions that don't warrant expensive trials but are still beyond the reach of registries or real-world evidence?
SH: Could you give us an example of such a mid-level question?
SP: Sure, questions like quantifying unmet needs in patient populations using existing therapies. This requires an active process that maybe informed by a clinical study, but there are confounding factors. Traditional methods like focus groups have their limitations due to their small sample sizes. Real-world evidence doesn't offer a clear answer, but the results could support market access negotiations.
Another example could be understanding the drivers behind medication adherence. This is hard to answer with real-world evidence, and it's not worth a clinical trial. Other methods have their downsides. The rise of large language models, like the current ChatGPT trend, has piqued interest in the pharma industry because they may offer new ways to answer these mid-level questions, although there are questions about their efficacy and application.
What excites you the most about the future of research?
SH: For our second question: "What excites you most about the future of research?"
SP: One aspect of research that excites me is the evolution of trial designs. While the methodology of trials hasn't drastically changed, there's been a group of individuals advocating for decentralized trials.COVID-19 and other factors have caused a surge of interest in this concept. Despite being a relatively small segment, decentralized trials offer many advantages like increased access, diversity, and reduced patient burden, among others. There are certainly challenges associated with this method, but overall, it presents a promising development for the industry.
Another thrilling change is the emergence of new technologies and tools to streamline trial processes, which have remained stagnant for years. In the past five years, several companies have come up with electronic data capture systems, e-consent systems, and patient management systems for trials. For instance, Seascape, a company from the first PharmStars cohort, developed an impressive tool that aggregates data from various databases into a single Excel-like file. This tool addresses a recurring challenge faced by trial managers, who spend countless hours gathering data to answer recurring questions about recruitment, patient enrollment, and other metrics. Tools like this, which enhance efficiency in trials, are undoubtedly a positive development.
From the perspective of medical device software, the kind of products being tested in trials right now is genuinely fascinating. If you go through the study titles on clinicaltrials.gov, you'll come across projects that seem straight out of science fiction. They're developing disease diagnoses using images, voice data, cough data, and wearable data. For instance, there's a company, GPx, working on a bloodless blood test linked with proBNP, a heart failure marker. All of these innovative projects being tested are undeniably thrilling.
Wave the magic wand...
SH: Our third question: "If you could wave a magic wand, what do you think you would change?"
SP: I believe this might sound rather unexciting, but I would greatly increase the amount of cross-functional, cross-team conversation and knowledge exchange. We often see clinical teams that don't have deep knowledge about data science, and data scientists don't always put forth efforts to explain to the clinical teams how products or algorithms are built.
Consider the example of study datasets. Theoretically, these are the cleanest types of medical data available, yet by traditional data science standards, they are still quite messy. Data scientists might approach a trial dataset with a uniform script, not considering the nuances of individual patients or subjects within the data set. They run their scripts across the entire dataset, disregarding the existence of various subgroups based on clinical factors. The clinical team, however, understands these nuances, but often this information doesn't get shared with the data scientists.
Furthermore, product teams may not fully understand the regulatory aspect, leading to issues when they begin building a product. They might design a product to be the best for the end user or patient, but in doing so, they might introduce labels and claims that can't pass the regulatory process.
Hence, there needs to be an ongoing conversation across these teams to ensure a smooth product development process. Currently, this kind of dialogue seems to be largely missing from many companies. I believe a change here could significantly improve the way we approach the development of medical products and software.
Latest Articles
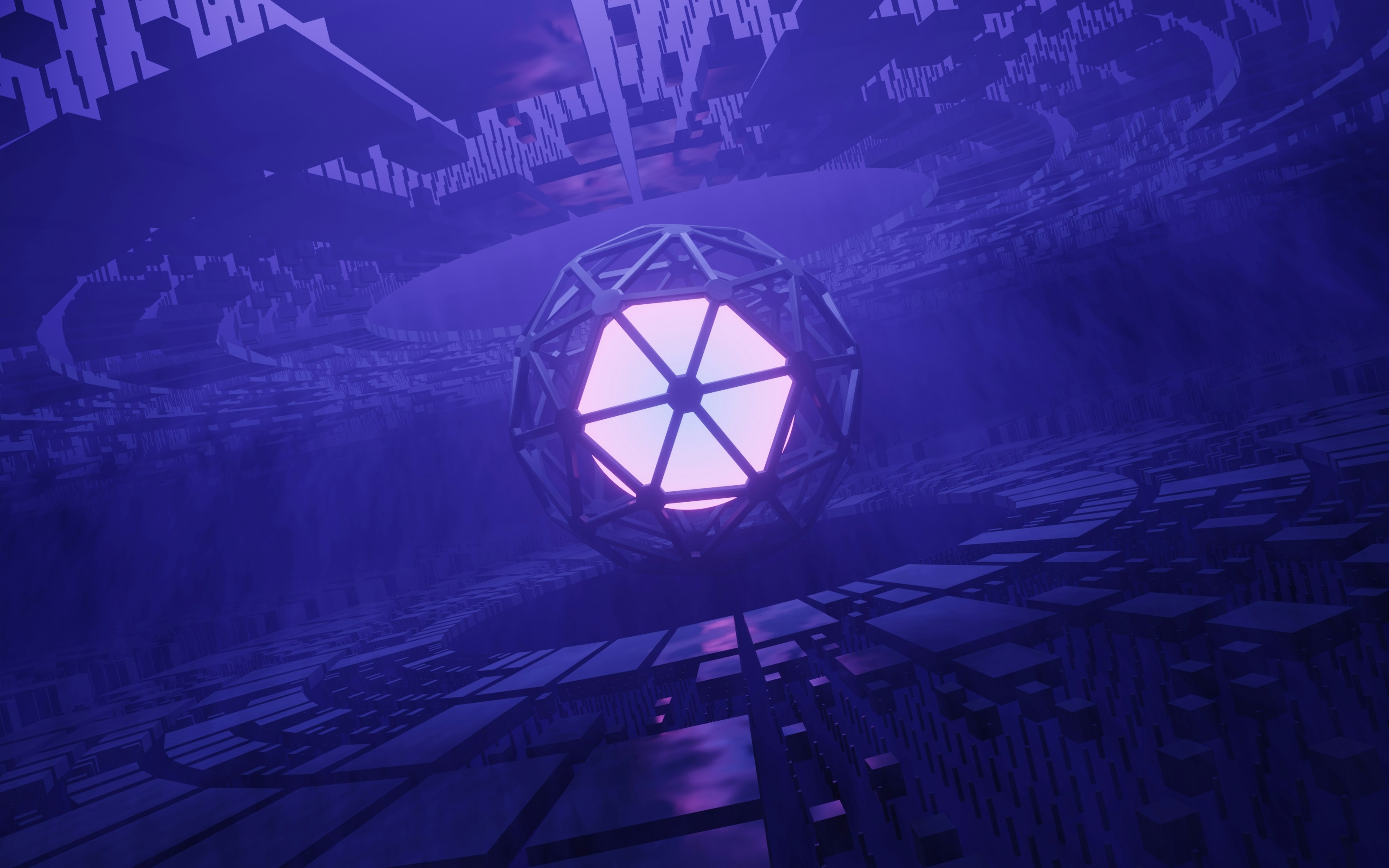
Understanding Large Perturbation Models
A brief, layperson's introduction to Large Perturbation Models (LPMs), a new tool in the drug development toolkit to simulate vast numbers of experiments digitally