Discussing Data-Driven Investment with Brendan Guerin
Written By:

In this month's Discussing Data Science episode, I talk with my co-founder about his background in private equity investing and his transition to Prism.Bio.
You can watch the video below or on Youtube. But if you'd prefer to read, keep scrolling. The complete transcript (edited for length and clarity) is below.
Transcript
Spencer Hey (SH): Hello! My name is Spencer Hey. I'm the co-founder and Chief Science Officer at Prism.Bio, and welcome to Discussing Data Science. My guest today is Brendan Guerin.
Brendan is my co-founder and the CEO of Prism.Bio. Before founding Prism, Brendan was an analyst at Origami Capital, a private equity firm based in Chicago, where he was particularly involved in their biotech portfolio.
Brendan is also a partner at Argos Partners Holdings, an investor group that focuses on pet boarding and daycare facilities, as well as a personal investor and advisor to a handful of other startups and small businesses. And all this at the ripe old age of 23!
Welcome, Brendan, and thank you for joining me today.
Brendan Guerin (BG): Thanks for having me.
SH: Why don't we start with your work as an investment analyst. In particular, it would be great to hear about the use and value of data in that role and some of the challenges you face. But it would also be great to hear the story, and hear more about what you were doing in that space before creating Prism.
BG: Absolutely. I started investing at a pretty early age and got really fascinated with the public market. I actually got a book on investing when I was 10 years old and became very obsessed with the field and very interested in becoming the best investor that I could be.
So I spent a lot of time thinking about what would be a good advantage to have as an investor. How could I really make myself differentiated in this field? And the thing that I always kept coming back to was just having a better grasp on the data than anyone else.
I was always a very math oriented person. In fact, I originally wanted to be a mathematician when I was younger. Eventually I decided it would be more interesting to do something more applied. But it was the application of math—or more specifically, data and evidence—that seemed like the way I could make the most sense out of the investment world.
I also started investing at a fairly interesting time. It was right after the Great Recession in the United States, and it was a time where you started to see a lot of folks doing data-driven investment. The traditional stock picker was fading into the background (these were the people that were trading "by hand," you know back in the days where the New York Stock Exchange had all these traders in the pit) and were getting replaced by computers and algorithmic, quantitative trading.
So I knew that I had to have an advantage as an investor if I wanted to really differentiate myself. I started to spend a lot of time thinking about data, and that was one reason why I started to focus on the life sciences investment vertical. Not only did I find that an interesting space to read about, I also had some early investment successes there. In fact, one of my best investments ever was actually in Abiomed—which was one of the biggest returners in the entire S&P 500 (it was like a 20x return on a medical devices company).
That was some good external motivation, but at the same time I found that biomedicine was an attractive domain. It's a field with a lot of complication, since you're not only looking at the individual dynamics of a company operating in the field but you also need to have an opinion on the research that's going on in the space and the companies that are surrounding it. It's an area that requires a little bit more elbow grease relative to consumer companies, where everyone tends to come in with the familiarity of what a company does. Biomed just had a little bit more nuance.
When I was considering investments in the space, I spent a lot of time reading the research that was published around a company, trying to understand their competitors, looking at data that was filed with the FDA and such. I was also trying to better understand how is this company positioning itself and how is it different than the competitors.
That was really the most important thing when I was making investments in the life sciences space: To understand the technology that a company was applying and determining if it was truly beneficial and better than the competitors. Because if it wasn't, then even if they had a good set of financial statements and they were performing well... my belief was that they would still lose in the long run. If you're not providing the best experience to patients and the people that are ultimately using the technology, then you can make some money, but it's not a long-term play.
So that was the hat that I wore when I was analyzing Investments both within my professional role and also within my personal role.
Building the Data Mosaic to Guide Good Investment
SH: One of the things that I'd be curious to hear you say more about is the sources of research and data that you used. You mentioned FDA, but what else did you rely on? Did your firm subscribe to journals or other kinds of databases? What types of data did you find the most useful?
BG: There were essentially, what I'll call, "three buckets" of data: (1) Data from the company itself; (2) public data; and (3) vendor data.
The data from the company itself was really an in-depth view of the financials, all the board decks that they were presenting internally, additional data that our firm had requested. We were doing private investments, so you can request a lot more data than a public investor can. But I think that's not actually the most interesting bucket to focus on. I think the interesting ones are the other two.
With public data—we did have access to journals and newsletters, and I was always closely following the news and subscribed to anyone that was publishing on business development and investment opportunities in the life sciences space. So that was kind of background information in my head. But when we were looking at an individual investment, I would delve into public data sources like ClinicalTrials.gov, PubMed, and FDA.gov. Those were always a good starting point for me to understand the competitive landscape around a company.
From there, I would always go to competitors' websites, and especially if they were a public company, there's a bunch of things that they are required to disclose. So that was other information that was really interesting to look into.
As an investment fund, we were also in the business of paying for a lot of additional sources of data, because if that helps us avoid a mistake, then it's just a minimal investment relative to the to the cost of a bad investment. We would regularly purchase vendor data sets, but those always depended on the specific opportunity.
So to summarize: We would start off with the same data sources in the public domain and from there we would go and find out who had the additional data that we need.
For example, we were looking at an antibody discovery platform: I spent a lot of time researching in the public data, then we brought in a very well-known life sciences consultant to help us understand the competitive landscape (using their proprietary data). This consultant had a lot of experience in the domain, so they had a nuanced view of the technology in that space. We purchased data sets from some other well-known vendors in the area that could provide us with good market share data, sales data, purchasing information, etc.
Then my job was really to take all this source of information—and we would always have these giant files of data that would sit on our company drive—and synthesize it.
One of the best investors that I know described the investment process as building a mosaic. It's thinking about every facet of a given company and building your mosaic piece by piece, to eventually understanding the holistic picture. Some stuff you find is going to be good, some is going to be bad and make you think it's a worse investment. But at the end of the day, what we were trying to do was build the most accurate mosaic that we possibly could and then use that to inform your decision making.
And that's why data was so important to me and my role. I knew that there's a lot of external factors that influence whether something's a good investment, but if I build the most accurate picture that I can, then at least I can rest easy knowing that I followed the right process and that I made the best decision that I could at that time.
SH: How long did that mosaic-assembly process typically take? How long from mosaic to decision?
BG: At every firm that I've worked at, it's a multi-month process. It really depends on the investment opportunity. I would say for Origami, the life sciences investment opportunities would take a little bit longer just because there's a little bit more complexity and you really want to understand.
Origami is not life a science-focused fund. We were a generalist fund that would invest in life sciences opportunistically. So we wanted to make sure that we understood every crevice of the investment opportunity. I think an average timeline, to just throw a number out there, was probably something like two to four months. And this would culminate in a giant, 100-page slide deck that goes into depth on every bit of what is this company, what does it do, how does the technology stack up versus legacy approaches, how does it stack up to the current set of competitors that might be trying to encroach in on the market, who's the management team, etc.
This would be paired with a very in-depth financial model that we would build as well. Truly a multi-month process that would require a lot of going back and forth. So we always did this in a phased approach where you put together the minimum amount of research that gets you to where you can understand the basics of the opportunities. Then we would look at all the opportunities that had come across our desk in a given week. Then we would decide which ones to focus on, and give people the opportunity to raise questions.
I would always have a list of questions that I would draft alongside building up the mosaic materials, where I could say, "Here's everything that I know. Now what's the additional stuff that I need to look into?" I would also then add in other people's questions as they were asking them, and then use all of these to guide the work for the next phase.
So I would do a little bit more research, we would spend a little money, perhaps we engage experts. That's actually another kind of vendor data set that we would think about: We would always talk to 5-7 experts in the space and get their opinions on the market.
But we're always building towards that full investment pitch and that complete investment model where we can really see and understand all the different pieces. That giant deck was really the living research artifact—the mosaic-in-progress—because we're constantly adding to it up until that final point where you make a go or no-go decision on that investment.
SH: Were there any steps in this process that were especially challenging; where you'd like to tear your hair out?
BG: It's a fairly manual and and painful process just across the board. You've probably seen those articles on the average amount of time that investment bankers or analysts at private equity or hedge funds spend in the office. I think a lot of that comes from the fact that this process is very custom per investment. We weren't a quantitative fund where we're building out a series of algorithms that are going to judge and dictate whether we think something's a good investment or not. We were focused on individual analyses of companies and opportunities. For us, this was always, almost by definition, going to be a very manual process. It was going to require a lot of time spent deep in research.
So I would say that this was a taxing process. When you finish the investment, I remember, it was always like a big sigh of relief. It's like: "Okay, that was a big sprint."
For me, the most complicated parts were synthesizing data. Whereas I always liked the financial modeling part. That was always a piece for me that was fairly standardized. You have to build out the model to make sure it accurately reflects the operations of the company, but that was a piece that I was quite familiar with because of my background.
I think an area that that I lost sleep over was understanding the research that underlies these companies. I've been investing in the Life Sciences for a long time, but I am not a PhD biologist. I don't have that sort of background and so I would try to approach it with a degree of humility. As someone who not an expert in this space, trying to read through the research literature required a lot of time. I was always wanting to make sure that I wasn't missing any big pieces. And so not only was I reading about the company, but I'm also trying to read publications and other sorts of primers on the technology that we were investing in. That was a lengthy process because I believe you shouldn't invest in things you don't understand.
Founding Prism.Bio
SH: So there you are, grinding away at researching biotech Investments, loving the financial modeling bit. You're putting in big hours creating these investment mosaics, but you're making six figures right out of college. And yet, you're thinking: "Not good enough. I want something more." Because that's not what you're doing now. Now you're running Prism with me.
So let's talk about that process: Maybe a little bit about how you and I met, but also what inspired you to want to take that step.
BG: I remember the exact day where I started thinking about the opportunity that we're working on today. It's very clear because that was a day that I read your STAT News article.
Just for the background and for everyone that's watching: Spencer published this article in STAT News that blew up, went viral, and was on the front page for like a whole week. The article was centered around the clinical trial portfolios of the top 10 largest pharmaceutical companies and understanding all the disease areas that they were active in, what their trial portfolios looked like, and just a variety of data related to that.
This was fascinating. I remember just being completely awestruck by the visualization: It's just this extremely detailed and long visual that helps you understand the top 10 pharma companies and what are they doing with their time. I'd never seen anything like this.
I would listen into the earnings calls from these companies and they have their own view on where they sit in the market. But never do they have a holistic view like this. Not ever.
I remember just being awestruck and just pausing and really thinking about this and probably read that article three or four different times. And then said to myself: "Well, I'm just this investment analyst, but let me reach out." I just wanted to talk with you, because it was one of those things where it was just kind of sticking in my brain.
So I reached out to you and we ended up meeting and discussing the opportunity. And one of the things I was really struck by was the similarities in the work that you and I did. Even though it was two very different careers, I think at the end of the day, we faced a lot of the same challenges; a lot of the same pain points. Most prominently: How do you synthesize complex data and come up with a clear and accurate picture of what's going on?
I was always working towards this kind of clear picture with these investment pitches, but the data and charts and all that were always static; they were always these point-in-time observations. Not being in the data science field either, there were limitations in the way that I would analyze things. So I remember thinking: "Wow, this is a massive opportunity."
For me, what I think it represented was the original pitch for Prism that we went out with: The Bloomberg for Biomedicine. I remember thinking that there seemed to be a lot of similarities in the trajectory for how data functions in the life sciences field and how it functions in the financial industry.
The Bloomberg terminal was this revolutionary product and technology that I think changed forever the way that you made money as an investor. There was a time in finance, when access to data was a problem and if you were reading 10Ks, you were requesting it from companies and you would have proprietary information that other folks didn't have.
Bloomberg standardized all this information and put it in one place. I don't want to say that it's pure access because it's an expensive product, for sure. But there are others, like Yahoo Finance, that have really replicated some of they key modules of the Bloomberg terminal.
But Bloomberg really revolutionized it, and I think all of a sudden, it changed the game from "Who can gather data" to "Who can make the best sense of the data". That was a fundamental shift in what made an advantage for an investor.
I think that we're in the early innings of a similar shift in the life sciences data world. Life sciences data is, of course, a lot more complex than what we see in the financial ecosystem. If you're looking at the P&L for a bunch of public companies and inputting that into Bloomberg, that's extremely valuable data, and there's a lot of qualitative data as well in just the general financial investment world. But I think you see a very rich, very different type of data in the life sciences world, and this has so far frustrated attempts to just put all that information out there and tie it together in a way that makes a lot of sense.
That's really what interested me the most about the Prism opportunity, as I saw this vision in the STAT News landscape article as a revolutionary idea to help the life sciences data start to look more similar to what we see in other industries. Because I think once you get to that point, where everyone kind of has the same access to data and people can really get their arms around it, there's just a lot more interesting work that goes on. And that to me is the starting point for a much richer and more vibrant life sciences ecosystem, where there can be a lot more progress; a lot more knowledge generation and insight generation.
The Bloomberg Terminal for Biomedicine
SH: Let's then pick up on exactly that point: The "Bloomberg for biomedicine"—which you've already described beautifully—is where Prism started. We ran with this idea and you pitched it a competition at Boston College as you were finishing up your undergrad. And what happened?
BG: The reception was great. I not only pitched it at the Strakosch Venture Competition, I also spent a lot of time talking to other friends and mentors in finance about the idea to get their feedback and input. I think everyone I spoke to saw this as a potentially transformative technology.
I used that feedback and momentum to put together the initial wireframe and we went to go pitch at Boston College. We ended up winning first place in that competition—all based off of this initial wireframe of the idea. I think it was just something that was tantalizing and that people were able to relate to. I think a lot of folks know what a Bloomberg terminal is, but don't know as much perhaps about the challenges of data in the life sciences unless you're in that world. But I think people saw the potential and they understood why it was important.
So we ended up winning first place, winning the initial check that we used to incorporate the company, and we received an invitation from SSC Venture Partners to apply for their accelerator program.
All of a sudden we had investor interest, where it was like: "Hey, this is a really interesting technology. Have you thought about pursuing this full time?" And I remember that was an exciting conversation, because, at the time, Prism still felt like just an idea I was exploring. But then this initial reception helped me understand the incredible potential here, and made me feel like: "Let's put this into practice. Let's get this out into the real world." And that was a very exciting moment.
SH: Part of what's interesting about this story is that "Bloomberg for biomedicine" is not how Prism brands itself today. If you go on our website, for example, we don't say "Bloomberg for biomedicine" anywhere. Why do you think that is?
BG: I think we started to see as we were going out into the market that the opportunity is even bigger than perhaps what originally we were thinking about. When we first started out, I was thinking about how do we arm the life sciences investor with the data that they need to make good investment decisions—which is a pretty specific use-case.
At the beginning of Prism, we had a lot of conversations with folks—whether that was biotech or VCs, whether that was folks that worked in pharma or folks in academia. We really spent a lot of time speaking with people. I think what I started to realize, and what we as a company started to think a lot more about, was the fact that there are more people that need access to a product like this. It's not just specific to the investor group.
So the "Bloomberg for biomedicine" framing resonates well with investors. You talk about "Bloomberg for biomedicine" and they pretty instantly get a picture in their head of what that would entail. When we're talking to folks in the drug development space—whether that's pharma companies or biotech companies—that framing doesn't resonate quite so well. But I think that's where a lot of the opportunity lies.
And I think one of the things that we find really exciting about the biopharma vertical (in contrast with the investor vertical) is that biopharma is an opportunity to arm folks with the right data who are not just funding the trials, but are instead the folks initiating the trials and running the research. They are the ones that own the assets. They're the ones trying to move those assets through the development pipeline.
That is why pharma may be the more interesting opportunity for a mission-driven company like Prism. We want to really make a difference in the lives of patients and research participants. Focusing on the biopharma space seems like a more direct way that we can drive real change for people. And what is more, the life sciences ecosystem is so complicated, and there's so many different parties involved, that looking at pharma as a place to start off with our product is extremely interesting.
So that's why pharma has ended up being a big focus for us in the early days. And I think that's been highly impactful. I'm really excited about the work that we've done there. There's a lot more great things to come, but I think that's been one of the biggest reasons why we've shifted away from the "Bloomberg for biomedicine" towards the pharma and data science framing that we have today.
What are the biggest data gaps in the field?
SH: Why don't we shift now to our three questions. So question #1: What do you see as the biggest data gaps out there in the field?
BG: Going back to wearing my investor hat: When I'm building my mosaic, I'm always thinking about where are there gaping holes. We spent a lot of time talking to the management teams of companies, talking to experts in the field, doing our own analyses—identifying where there were holes and filling them. Generally, that was a pretty straightforward process. Someone mentioned something, we'd dig into it, spend a bunch of time researching.
I think where we had the biggest gap was actually in linking all the data together. This is another way in which the mosaic metaphor is helpful because we'd have these little sections of the mosaic that were sitting on their own—like little islands of analysis and data. And the question I'm always asking myself is: What am I missing?
PowerPoint presentation are really the knowledge currency that exists in the investment world but those are not super conducive to understanding links between data. If you just think about what a PowerPoint presentation is— it's very mosaic-like. It gives you one section of analysis. Flip. One section of analysis. Flip. And so on.
I spent a lot of time trying to think about how I should put these pieces together. How do the individual slides or sections influence one another? How do I understand the way that a company is positioning itself? Then how does that inform my analysis of their competitors? And how does that inform my analysis of the people that are trying to break into their space? How does the financial performance of this company over the past five years impact their product development timelines?
I think all of that synthesis is extremely complicated, and so I was always wanting to understand these connections better—to not just build a mosaic, but to really tie it together in a way where you could see connections between these different islands of data.
Another big gap was the human or expert opinion piece of the mosaic and figuring out how to overlay that into the way that we built our analyses.
We were always talking to domain experts, because getting those opinions can be so valuable. But sometimes those opinions are not data driven. Sometimes the expert that we're interviewing is biased or just going off of intuition. Figuring that out, and figuring out how to weight those opinions, was always complicated. It's just a very different kind of data when you're looking at the transcript from an interview, or even a public company's meeting transcript. It's hard to put that into a financial model. So I spent a lot of time thinking about that as an investor and trying to get better at it—but it is still a huge area of complication.
What excites you the most about the future of research?
SH: You've touched on this a little bit already when you were talking about the opportunity with the "Bloomberg for biomedicine", but what excites you the most about the future of research? Is there some new development that you see happening that makes you think, "This is going to be transformative when it comes down the down the pipe."
BG: Definitely. One of the opportunities that I'm really excited about is seeing more "living research". I'm not only thinking about Prism here. I think that we're starting to see a lot more connections between data sets; a lot more integrations in the ecosystem. We're seeing a lot more folks build platforms with the intent of communicating with other platforms. I think that this is going to be extremely transformative.
When I was working as an investor a lot of times what we were faced with was either we had a very specific data sets or software solutions that really were custom built to do just one thing. Those tools could be helpful, but by definition, it just added to the islands of data that we were talking about above with the data gaps between them.
We also worked with consulting firms. And that was great because they were a little bit more holistic in the way that they thought about investment opportunities. But at the same time, they didn't have the same skin in the game that we did as the investor. They weren't getting paid based off the failure or success of the deal, and so there were always some skepticism with regard to their analyses.
Where I really see opportunity is in the middle space between these two—the stand-alone data sets and tools, on the one hand, and the custom analyses of a consultant, on the other. This is where see the companies or products in the living research space: They are pulling together different sources of information in real time.
I think that's extremely interesting from an investor's perspective because it can transform the static PowerPoint presentation—the final result of our investment research—that could only ever be just a point-in-time analysis whose insights had to be held in your head. This can be transformed into something "living and breathing" and structured in a way that it provides continuous value over time.
I think as we're starting to see more and more of that—complex mosaic-like analyses that can evolve and update themselves—we're also going to see better decisions being made. We're going to see winning companies get funded; we're going to see companies that aren't developing interesting technology not receive funding. So I'm just excited about more evidence-based decisions being made, because that benefits everyone in the ecosystem.
SH: I'm curious to pick up on something there. There has been talk about living evidence reviews in academia for a while now. Going back to the genesis of Prism: That was one of the things that you and I initially connected on—we both saw opportunity in the fact that the traditional systematic review is considered the gold standard for evidence synthesis, and yet, it takes a really long time to do right. Experts spend months or even years doing this work. And then when it's finally done, it's written up in a manuscript that has to make its way through the peer review process before the ecosystem can derive any real benefit.
So it seems strange and suboptimal that what you get out of the gold standard evidence synthesis process is a static document that, in the best case scenario, is the answer to a question that is 12 months old. For some questions, that might be good enough. But it's easy to see how this method will simply never keep up with the pace of new research and new data and new questions that need answers—and may need answers more quickly!
Academics have been talking about this problem for at least 10 years. It seems to me that we're not really seeing much of a shift in that realm. So I'd be curious to hear you say a little bit more about where you see this shift happening towards living reviews.
(Obviously this is a part of what Prism is doing with our academic clients, but outside of that.)
BG: Sure. When we were doing investment research, if we could find a reputable systematic review that was relevant to the space, that was like the Holy Grail. That was really exciting—even if it was old. A good point-in-time observation that we could factor into our decision-making process was always valuable.
It's funny because even though the processes and intended uses are very different, it always struck me that the final output of a systematic review and the final output of an investment pitch are quite similar.
With the investment space, that's where I see a move toward more living research. I think we're seeing more and more people starting to think about, for example, tracking competitive universes. Even if I'm making my investment decision at time X, I can now start to track my competitive analysis—and even review my decision or decision criteria—over time.
I think we're starting to see more investment tools that have really been built around the idea that you can, and should, continue to follow a space that you've invested in (or are just interested in). The idea is simply to minimize the amount of time that exists between research artifacts.
I think what you're going to start seeing is a lot of living investment analyzes—living pitch decks where you've got your point-in-time perspective, but then you can click to drill down and see the living data set. Or perhaps you'll even see products where you can see updates in real time.
I think this will be really important because, as part of the investment in portfolio management piece, you want to understand the changes that are going on within the ecosystem. I think what we see now is updates on LinkedIn, or you're following the news, or you're going to have your quarterly board call or something like that.
But I don't think that people are yet approaching the tracking or updating piece of the investment process with anywhere near the same level of rigor that you approach the initial investment decision. And there's a lot of data now to suggest that when you exit is in some ways just as important as when you enter.
So I think that that's just an important piece that needs to be more involved. To make good investment decisions and really maximize ROI, I think we're going to see firms increasingly look to living research and analysis tools.
That's also one of the nice parts about the investment sphere: If it truly is the case that you produce better investment returns when you factor in these living analyses, then those firms are going to produce better returns over time. People are going to wonder what are they doing over there where they're getting these returns. And then you're going to start to see other people shift their behavior to copycat and try to improve their own investment returns. So that's one part I really like about the investment space is I think best practices tend to take hold pretty quickly because it's like if it truly is the case that you're generating better returns, everyone wants to know why and try to copy that for themselves.
Wave the magic wand...
SH: All right! Question #3, Brendan: Wave the magic wand—What would you change about research for the industry?
BG: I think for me, a lot of what I'd like to change concerns human dynamics. To me, that stuff is the hardest thing to change. By contrast, if you look at the progress that we've seen on the technology front for all these issues: We're looking at new living research tools; looking at the vast amount of data we have access to... There's never been a time like this ever before.
What has moved slower is human attitudes, and a lot of the dynamics around the human element of adopting or using more/better data or these new tools. So if I could wave a magic wand, I would want everyone to start taking more of a data-driven attitude.
I would also want people to play nice in the sandbox. I think there's been opportunities in areas like, for example, the travel industry: As a group, travel has done really well with sharing great data. If you look at like Expedia or if you look at the hotel industry and the metrics that they share with one another: It's actually fairly routine where people will go out and they will compile together data. One company will say, "Hey, what's your occupancy? Help me understand what your average booking rate has been," and all this stuff.
It's funny because if you think about the perspective of one single hotel: Do they want to share all this information about performance? Even if the data is anonymized? You might originally think, "No".
But it turns out, there's all these benefits that occur, not only for us consumers, but also for the hotels, if they start to share that information. When you start to do that, all of a sudden you have a richer picture of your business. You can see things like: "Okay, I am not getting the level of occupancy; I'm not getting the pricing that I want. So what do I need to change to make sure that I'm competitive in the ecosystem?"
That's just one case study of an industry that's benefited from data sharing. But I think that people are still quite protective of their data in the life sciences ecosystem—and in some cases, rightly so (e.g., private patient data). But I think the more that we see people sharing information, the more that we see people wanting to integrate with other data sets and build that into the way that folks are creating products in this world—I think we're just going to see a lot better results.
In order for us to realize the benefits of all of this data, we need to have a better attitude collectively around sharing that data, around working together, and around making data-driven decisions. Whether it's the investment world, whether it's pharma, whether it's academia—I think we still see a lot of decisions based off opinion and intuition and we see cases where that kind of decision-making leads to bad results.
So what I would really want is for more people to take dispassionate approach and see how sharing data can help to maximize value for the total ecosystem. I really do think the way that efficient markets should run is where everyone's sharing the information and people are competing to become best-in-class, rather than trying to gain a competitive advantage by withholding data.
While you can win in the short term by holding back data and information, I don't think that's the the healthy way to function and I don't think that's where we're going to end up long term. I think it's really just a matter of time before we start to see an explosion of progress because more folks are willing to share and play nice in the sandbox.
Indeed, we've seen that firsthand at Prism, through our involvement with groups like The Pistoia Alliance, which is all about pharma and biotech competitors working together to solve problems in a pre-competitive way. I think trends and initiatives like that are just going to increase and as we start to see more and more activity in that pre-competitive space, we're going to start to see faster progress as a result.
Conclusion
SH: Brendan thanks so much for having this conversation with me today. You and I talk all the time, but it's really nice to dive back into some of the history and hear more about your expertise in the investment space.
BG: Thank you. This has been a fun walk down memory lane and thanks everyone for watching.
Latest Articles
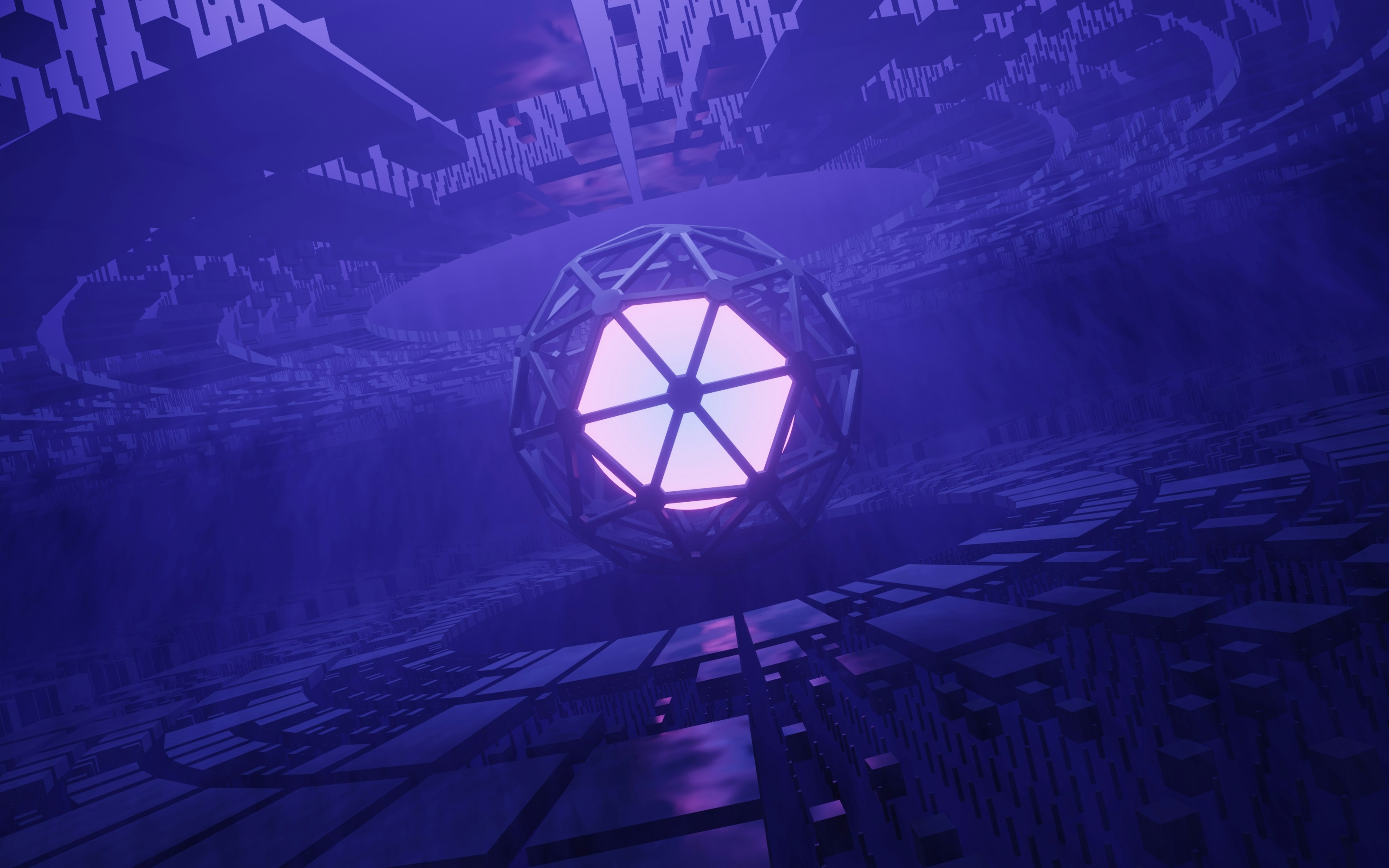
Understanding Large Perturbation Models
A brief, layperson's introduction to Large Perturbation Models (LPMs), a new tool in the drug development toolkit to simulate vast numbers of experiments digitally